M.Sc. Andreas Bott
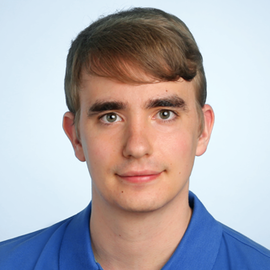
State estimation in distributed heat networks
+49 (0) 6151 16-21720
fax +49 (0) 6151 16-21712
S3|10 303
Landgraf-Georg-Str. 4
64283 Darmstadt
Research Interest
- Modelling of district heating networks
- Probabilistic State Estimation
- Flexible Operation and Scheduling of District Heating Networks
- Machine learning and data analysis in the energy domain
Research Project:
Space heating accounts for more than 50% of the final energy consumption in private housing. Therefore, means to provide CO2-neutral heat is an important step towards a Carbon neutral future. District heating networks of the 4th generation can provide this energy in densely populated areas and cities as they connect decentralized heat sources such as industrial waste heat and large-scale heat pumps with flexible consumers and heat storages. As heat can be stored cheaply and easily, and the heating sector is coupled with the electrical sector via heat pumps and combined heat and power plants, flexible operation of district heating grids can also help balancing the fluctuating feed in in the electrical grid by renewable energy sources.
Traditionally, district heating grids were used as distribution grids with a low number of feed-in points and easy but robust control schemes, that did not utilise its flexibility potential. In the project MeFlexWärme new methods for the flexible operation of district heating grids are developed, focusing on an efficient scheduling of different heating sources and on providing incentives for participants to adapt to the grids state through a local energy marked.
Network transparency is key in enabling a flexible grid operation while ensuring the supply of all customers. Measurements are sparsely placed in district heating grids, such that the temperatures and pressures have to be estimated based on the available information as best as possible. Statistical methods and machine learning approaches are utilised to develop new models for this state estimation.
Open theses
Supervisor: Andreas Bott
Earliest start: immediately
Type: Master Thesis
In order to reduce CO2 emissions, district heating grids will have to utilise decentralised heat sources such as large-scale heat pumps and industrial waste heat. Operating these so called 4th generation heating grids requires the development of new algorithms. Classical approaches are computational costly, due to the complex non-linear behaviour of district heating grids. AI based algorithms on the other hand are promising to deliver reliable and fast results.
This Thesis should investigate the optimal dispatch problem, i.e. the question which plant should produce heat at which temperature, such that all grid constrains are satisfied. This problem resembles a mixed integer, nonlinear optimisation problem, which is difficult to solve. The goal of this thesis is therefore, to learn the solution of the optimal dispatch problem directly based on the current demand situation.
The true cost function and boundary conditions are (practically) not differentiable. This leads to different approaches, which can be analysed within the scope of the Thesis:
- Use Reinforcement algorithms to train the networks on the true loss function. As evaluating the cost function is still costly, this would require pre-identifying critical points to reduce computational costs.
- Approximate the true cost function and boundary conditions with a differentiable model (e.g. another neural network) and use this ass a loss function during the training of the dispatcher
- Learn the optimal dispatch and the description of the district heating grid simulations by employing a physics-aware Loss function
Supervisor: Andreas Bott
Earliest start: immediately
Type: Bachelor Thesis
In order to reduce CO2 emissions, district heating grids will have to utilise decentralised heat sources such as large-scale heat pumps and industrial waste heat. Operating these so called 4th generation heating grids requires the development of new algorithms. If a grid has multiple feed ins, at one point the water from different supplies has to mix. Identifying this point is of special interest, as it is most likely to violate grid constrains, e.g. for the water to be to cold or the pressure to be to low. This thesis analyses a special measurement arrangement in order to identify this mixing point.
We assume to a grid segment with multiple uncertain demands. The position of the mixing point depends on the realisation of the demand. We assume, that the pressures and the mass flows at both inlets to the grid section are measured. This setup is especially interesting, as it allows to formulate the power estimation problem as a quadratic optimisation. The thesis should formulate and evaluate the results of this optimisation problem to evaluate the measurement setup.
Supervisor: Andreas Bott
Earliest start: immediately
Type: Master Thesis
Die Wärmewende bedeutet für den Fernwärmesektor eine grundlegende Umstellung. Statt wie bisher die Wärme aus wenigen zentralen Heizkraftwerken zu verteilen gewinne dezentrale, fluktuierende (Ab-)Wärmequellen zunehmend an Bedeutung. Dieser flexible Betrieb erfordert neue Algorithmen um eine sichere Versorgung zu garantieren.
Eine besondere Herausforderung im Kontext von Wärmenetzen ist die relativ langsame Fließgeschwindigkeit des Wassers. Hierdurch kommt es zu einer erheblichen Verzögerung bis eine Betriebsentscheidung am Kraftwerk den gewünschten Effekt im Netz zeigt. Entscheidungen müssen daher vorausschauend getroffen werden und mögliche Entwicklung unsicherer Größen, beispielsweise die Entwicklung des Wärmebedarfs, vorab mitberücksichtigen.
Aus dieser Problematik lassen sich zwei Voraussetzungen für Netzmodelle ableiten.
1. Die Modelle müssen die Zeitabhängigkeiten und Laufzeiten korrekt abbilden
2. Die Rechenzeit muss relativ gering sein um in probabilistischen Algorithmen, z.B. in Monte Carlo Simulationen, eingebunden zu werden.
Klassische Simulationssoftware, wie Modelica, erfüllen zwar den 1. Punkt, sind aber zu rechenaufwendig für Echtzeitanwendungen.
In dieser Arbeit soll eine neuartige datengetriebene Modellierungstechnik reduzierter Ordnung vom Typ der neuronalen ODE angewandt werden, um ein genaues und schnelles Ersatzmodell der Simulationsmodelle zu generieren, das zudem einen geringen Rechenaufwand aufweist. Die Arbeit umfasst dabei einerseits die Konstruktion konventioneller Modelle in Modelica als Benchmark sowie zur Erzeugung von Trainingsdaten, andererseits auch Einbinden und Trainieren der datengetriebenen basierten Modelle in Python. Sie schlägt damit eine Brücke zwischen klassischer Modellierung und modernsten KI-basierten Ansätzen.
Short Bio
since 2020: PhD student at EINS
2016 - 2019: M.Sc. Energy Science and Engineering at TU Darmstadt
2012 - 2016: B.Sc. Physics at TU Darmstadt
Publications
[Journal]
Mario Beykirch; Andreas Bott; Tim Janke; Florian Steinke :
The Value of Probabilistic Forecasts for Electricity Market Bidding and Scheduling Under Uncertainty.
In: IEEE Transactions on Power Systems , 2024
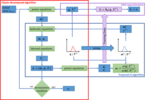
[Conference]
Andreas Bott; Steinke Florian :
Efficient Training Data Generation for Learning-Based State Estimation in 4th generation District Heating Grids.
In: 9th International Conference on Smart Energy Systems (SESAAU 2023), Copenhagen, Denmark, 2023
[Journal]
Andreas Bott; Tim Janke; Florian Steinke :
Deep learning-enabled MCMC for probabilistic state estimation in district heating grids.
In: Elsevier Applied Energy 336 , P. 120837, prePrint, 2023
[Conference]
Andreas Bott; Pascal Friedrich; Lea Rehlich; Florian Steinke :
Model Reduction for Heat Grid State Estimation.
In: 2021 IEEE PES Innovative Smart Grid Technologies Conference Europe (ISGT-Europe), virtual Conference, 2021
[Conference]
Andreas Bott; Alexander Matei; Florian Steinke; Stefan Ulbrich :
Methodenbaukasten für Flexible Wärmenetze der Zukunft.
In: Konferenzreader Digitalisieren, Sektoren koppeln, Flexibilisieren: Systemische Integration der Bioenergie und weiterer erneuerbarer Energien in Gebäuden & Quartieren, virtual Conference, 2020