M.Sc. Sina Hajikazemi
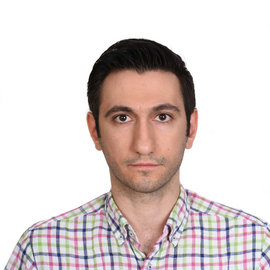
Adversarial attack on energy system models
+49 (0) 6151 16-21719
fax +49 (0) 6151 16-21715
S3|10 305
Landgraf-Georg-Str. 4
64283 Darmstadt
Research Interest
- Robust optimization
- Bilevel programming
- Parametric programming
- Sensitivity analysis
Research Project:
How much do the decision outputs of the energy system models depend on each set of input parameters?
Can big companies imperceptibly change the input parameters in order to change the decision outputs in a desired direction?
To answer the above questions, I am currently working on sensitivity analysis of the mathematical optimization based energy system models. The focus of my research is mainly on the bilevel linear programming models with millions of variables which is NP-hard in the general form.
Open theses
Supervisor: Sina Hajikazemi
Earliest start: immediately
Type: Master Thesis
Energy Planning Models (EPMs) are essential tools for simulating and guiding decisions about multimodal energy systems in specific regions or countries. These models provide strategies to meet future demands and environmental targets, which are often used by large energy companies to negotiate with the government and other investors. However, there is a concern that these profit-driven companies could manipulate the input data of EPMs to align with their interests.
This thesis aims to address this problem by formulating it as a bilevel mathematical programming model. The upper-level problem represents the adversary who attempts to alter the input data, while the lower-level problem corresponds to the EPM. While some algorithms can effectively solve the linear upper and lower-level form of this problem, more advanced and detailed EPMs require the use of binary variables, resulting in a much more challenging Bilevel Mixed Integer Linear Programming.
The student will review available methods for solving bilevel programming problems with binary variables and tailor these methods to the aforementioned adversarial attack, taking into account the size, special form, and other specifications of the EPM. The designed algorithms will be implemented, and the results will be interpreted. This study will contribute to developing robust energy planning models that are resistant to adversarial attacks, ensuring that decision-making processes are fair and transparent.
• Familiarity with energy planning models
• Proficiency in python programming
• Experience with mathematical programming languages
Supervisor: Sina Hajikazemi
Earliest start: immediately
Type: Bachelor Thesis
Energy planning models are essential for analyzing energy and climate policies at national and global scales. However, these models face various uncertainties, categorized into uncertainties in input parameters, such as future fuel prices, and uncertainties in the structure of the model, such as the complexities and constraints inherent in different technologies. While methods such as global sensitivity analysis, stochastic programming, and Monte Carlo simulation address parameter uncertainties, they often overlook uncertainties in the model structure. In addition, policymakers are faced with considerations outside the scope of conventional modeling, such as political feasibility, regulatory challenges, and the timing of actions. As a result, policymakers may choose feasible but suboptimal solutions due to the challenges of quantifying intangibles in energy optimization models. Modeling to Generate Alternatives (MGA), a technique borrowed from the operations research literature, is a valuable approach to address structural uncertainties inherent in energy planning models as well as uncertainties in input parameters. MGA efficiently explores the feasible region around the optimal solution and generates alternative solutions with maximum diversity. By providing a spectrum of viable options beyond the conventional optimal solution, MGA provides invaluable insights for policy makers. These alternative solutions shed light on trade-offs and considerations often overlooked in conventional energy planning models, enabling policymakers to make more nuanced and informed decisions amid uncertainty and real-world constraints. This thesis focuses on implementing this approach in a German energy transition model (see github.com/EINS-TUDa/CESM) and exploring the results and insights it can provide to decision makers. Project Tasks:
- Understand the Modeling to Generate Alternatives (MGA) methodology.
- Apply the MGA methodology to the German energy transition model using the Compact Energy System Modelling Tool (CESM).
- Investigate the outcomes of the MGA implementation and identify the insights it offers for policymakers.
- Evaluate the strengths, weaknesses, and obstacles associated with the MGA methodology. Prerequisites: Proficiency in Python programming.
- Gain basic knowledge of mathematical programming techniques necessary for basic optimization tasks relevant to energy planning models.
- Develop a fundamental understanding of energy planning models, including their components, basic methodologies, and applications in energy policy analysis.
- Learn basic skills in reporting and justifying the outcomes of energy planning models, including simple interpretation of findings and basic assessment of model validity.
Supervisor: Sina Hajikazemi
Earliest start: immediately
Type: Master Thesis
This master's thesis explores the potential of harnessing Large Language Models (LLMs) to transform user interactions with energy system models, with a focus on developing a Textual User Interface (TUI). The study investigates the interconnection between LLMs and Application Programming Interfaces (APIs) in order to facilitate a more intuitive and user-friendly means of engaging with complex energy system models. The research aims to bridge the gap between energy system model developers, decision-makers, and the public by providing a user-friendly TUI that enhances transparency and decision-making processes.
Research Objectives:
1. Examine the emerging role of LLMs in the future of user interfaces and their potential to transition from graphical to textual interfaces.
2. Develop a TUI that interfaces with Energy System Models (ESMs) via well-documented APIs.
Methodology:
1. Investigate and select appropriate tools and frameworks for integrating LLMs with APIs, drawing on existing examples and technologies, such as LangChain's Python Library for Use Cases with APIs (https://python.langchain.com/docs/use_cases/apis) and OpenAI's GPT Function Calling (https://platform.openai.com/docs/guides/gpt/function-calling).
2. Define use cases and questions that the LLM-based TUI can address to enhance understanding and decision-making in the context of energy system models.
3. Create well-documented API specifications using standard formats (e.g., OpenAPI) for a reputable energy planning model, such as "Open and Compact Model for the German Energy Transition," (available on GitHub: https://github.com/OCGModel/OCGModel).
4. Establish a functional connection between the API and LLM to develop a working prototype of the LLM-based TUI.
Significance and Contributions:
This research contributes to the evolving field of human-computer interaction by demonstrating how Large Language Models (LLMs) can be integrated with energy system models, providing an innovative approach to user interfaces in the context of energy planning and governance. The development of a TUI for ESMs enhances transparency and public engagement, while also encouraging model developers to improve precision and openness in their assumptions and methods.
By addressing these objectives, this master's thesis aims to facilitate better-informed decision-making in the energy sector, foster transparency in energy model development, and contribute to the broader discourse on the role of LLMs in future user interfaces and decision support systems.
Keywords: Large Language Models (LLMs), Textual User Interface (TUI), Energy System Models (ESMs), Application Programming Interfaces (APIs), User Experience, Transparency, Decision Support, Energy Planning, Human-Computer Interaction
Supervisor: Sina Hajikazemi
Earliest start: immediately
Type: Bachelor Thesis
Electric transmission grids are critical energy infrastructures in every country. Intelligent attackers may attempt to damage specific components of the grid to cause maximum load shedding, and grid operators respond by solving the power flow problem to minimize load shedding using the remaining intact components. This raises the question: How vulnerable is the grid to adversarial attacks?
This project focuses on implementing and understanding an iterative optimization algorithm proposed by Javier Salmeron et al. [1] for the Electricity Network Interdiction problem. The algorithm formulates the problem as a bilevel programming problem, where the attacker aims to maximize load shedding, and the grid operator aims to minimize load shedding through optimal power flow in the attacked network.
Project Tasks:
1. Implementation: Implement the optimization algorithm in Python, ensuring clean and well-structured code.
2. Documentation: Provide clear and concise documentation for the implemented code, explaining key functions and algorithms.
3. Testing: Develop and execute test cases to validate the functionality of the implemented code.
4. Version Control: Utilize Git for effective code management and version control.
5. Presentation: Prepare a concise presentation that explains the project's objectives, methodology, and findings.
Prerequisites:
• Proficiency in Python programming.
• Basic understanding of mathematical optimization concepts.
Reference:
[1] Salmeron, Javier, Kevin Wood, and Ross Baldick. "Worst-case interdiction analysis of large-scale electric power grids." IEEE Transactions on Power Systems 24.1 (2009): 96-104.
Supervisor: Sina Hajikazemi
Earliest start: immediately
Type: Master Thesis
AC Optimal Power Flow is a fundamental building block for several optimization problems of electrical power systems, such as unit commitment and optimal transmission switching. It is a nonlinear and non-convex optimization problem, and solving it to optimality is an NP-hard problem. In practice, this problem is solved using linearized approximations for computational tractability. However, linear approximations can lead to suboptimal solutions or solutions that are infeasible in the original nonlinear problem. With the introduction of stochastic energy sources into the grid, the need to solve the AC-OPF more frequently has increased.
A recent approach to overcome this difficulty is to combine machine learning techniques and optimization algorithms such as Lagrangian dual methods [1]. The learning model exploits the information in the similar states of the network and the optimization algorithm satisfies the constraints of the problem.
In this thesis, the student will conduct a comprehensive review of the existing literature on these innovative techniques. Subsequently, the most promising approach will be implemented and rigorously evaluated. This will be followed by a comparative analysis comparing the performance of the chosen methodology with alternative strategies. The study aims not only to investigate the potential of using these hybrid methods to solve the AC OPF, but also to shed light on the potential of combining deep learning and nonlinear optimization to address complex challenges in modern power system optimization.
[1] Fioretto, Ferdinando, Terrence WK Mak, and Pascal Van Hentenryck. "Predicting ac optimal power flows: Combining deep learning and lagrangian dual methods." [https://arxiv.org/abs/1909.10461]
Short Bio
2010-2014: BSc Civil Engineering, Sharif university of technology, Iran
2014-2016: MSc Applied Mathematics(Operational Research), Ferdowsi university of Mashhad, Iran
since 2022: PhD student at TU Darmstadt