Student: Tim Frieß
Supervisor: Allan Santos
Time period: 09/01/2022 - 03/01/2023
Type: Master Thesis
Die Optimierung von Versorgungssystemen ist zentraler Bestandteil im Untersuchungsfeld der energieeffizienten Produktion. Neben der Energieeffizienz tritt immer mehr auch das Anpassen des Strombedarfs an die Erzeugung aus erneuerbaren Energien in den Vordergrund aktueller Forschungsvorhaben. Dabei bieten Wärme- und Kälteversorgung einen großen Hebel bei der Energieflexibilisierung von Versorgungssystemen zur Raumklimatisierung in Produktionsbetrieben. Durch Einbezug aller Flexibilitäten kann die optimale Betriebsstrategie mit Betrachtung aller Verbraucher sowie interner und externer Einflussfaktoren für einen Zeithorizont in die Zukunft ermittelt werden. Im Rahmen der nichtlinearen mathematischen Optimierung ist es wichtig, möglichst einfache Modelle der Einzelkomponenten zu verwenden, welche dennoch mit hoher Genauigkeit die reale Anlagendynamik wiedergeben. Dadurch werden sowohl Rechenzeiten geringgehalten als auch die Skalierbarkeit der Systeme gewährleistet.
Zunächst sollen durch Anpassung vorhandener Simulationsmodelle mit Kennlinien aus Vorversuchen sowie physikalischer Modelle der einzelnen Systemkomponenten umfangreiche Datensätze erzeugt werden. Auf Basis dieser Daten sind unter Zunahme passender Algorithmen vereinfachte Modelle der Komponenten zu identifizieren. Diese Modelle sollen anhand realer historischer Daten parametriert und validiert werden. Die identifizierten Modelle sollen abschließend zu einer Optimierung des Gesamtsystems genutzt werden. Dabei sind für die gefundenen Modelle die Lösbarkeit und Lösungsgeschwindigkeit des Optimierungsproblems zu vergleichen.
Student: Andreas Steinmetz
Supervisor: Sara Mollaei
Time period: 06/01/2023 - 11/30/2023
Type: Master Thesis
Technological advances in information and communication technology, e.g., cloud computing, enable telecommunication operators to shift many energy-intensive computations and data traffic across various locations, so-called central offices. This project aims to address the economic aspects of demand shifting in these central offices. The scope of the project will include analyzing the company's current demand-shifting practices, including computation shifting from one central office to another based on electricity pricing and computation capacity, as well as shifting computations to a later time in the same central office. The project will use a combination of economic analysis and optimization techniques to find an economically optimal and robust solution for demand shift planning, taking into account both temporal and spatial demand shifting.
The work is conducted based on data from a large German telco company.
Student: Khalil Tounsi
Supervisor: Tim Janke
Time period: 08/01/2020 - 10/31/2023
Type: Project Seminars Bachelor
It is well known that model combination approaches, also known as forecast averaging or ensembling, can often improve the forecast accuracy. Liu et al. [1] report an improved performance for the task of probabilistic load forecasting using quantile regression averaging. Probabilistic forecasts improve over classic point forecasts by additionally reporting the uncertainty of a prediction, usually in form of a probability distribution. This thesis should first implement the approach proposed in [1] and then compare the proposed method empirically against other forecast combination approaches across several data sets. Prospective students should be interested in statistical modelling and machine learning and have some prior experience with programming languages like Python, R, or Matlab.
[1] Liu, B., Nowotarski, J., Hong, T., & Weron, R. (2015). Probabilistic load forecasting via quantile regression averaging on sister forecasts. IEEE Transactions on Smart Grid, 8(2), 730-737.
Student: Anonym
Supervisor: Martin Pietsch
Time period: 05/15/2023 - 10/16/2023
Type: Bachelor Thesis
emergenCITY aims at investigating fundamentals, methods and solutions towards the resilience of future digital cities. This includes the development of practical solutions for new requirements in crisis scenarios. In this context, we at the Mission eHUB are working on local solutions for resilience increase through decentralized energy islands. In order to mitigate the impact of crises, such as a prolonged power outage, the eHUB should be able to offer charging possibilities to the neighborhood/district.
Within the scope of this work, you will develop and construct a smart charging station. The focus should be on simultaneously providing power to as many people as possible and implementing different charging strategies. The charging strategies should, for example, distinguish between fast charging and partial charging in order to be able to react according to the circumstances of the crisis or the power availability of the eHUB.
A 3D printer is available for the construction. The design should be adapted to previous developments.
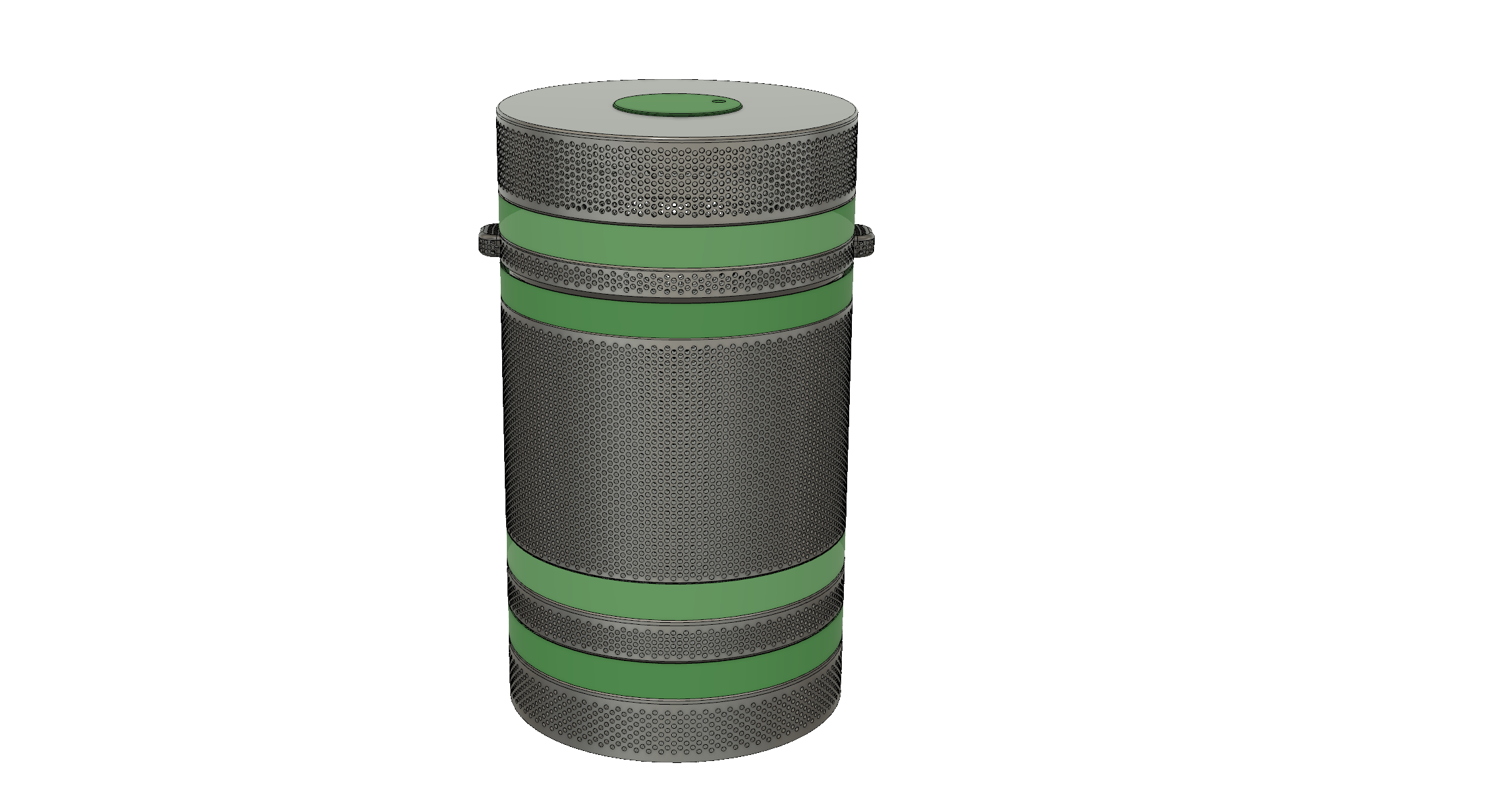
Student: Luis McMillan
Supervisor: Andreas Bott
Time period: 04/17/2023 - 10/16/2023
Type: Master Thesis
Decarbonising the heating sector plays an important role in achieving a low-carbon energy system. District heating grids can help in this regard, however, efficient controll and utilisation of modern grids requires in depth knowlege over the current grid state.
Determining the grid state is computationally costly, however, the exact solution of the nonlinear system can be approximated using Neural Networks. Graph-Neural-Networks seem especially siuted for this task, as they include spatial informations in theyr architecture. The aim of this work is to develope a GNN structure for district heating grids and to test different training scheemes.
Student: Nora Filipczak
Supervisor: Kirill Kuroptev
Time period: 01/01/2023 - 06/30/2023
Type: Master Thesis
Disruptive technologies like the “Internet of Everything” and the “Metaverse” result in enhanced traffic in the information network nodes, also called central offices, that connect customers to the Internet. As this traffic increases, the energy consumption and CO2 of the nodes do so, which gives rise to the need to assess the technical infrastructure – e.g., cooling, power supply – of the central offices to cope with environmental and economic challenges.
The thesis aims to formulate and solve an optimization problem regarding relevant objectives - e.g., implied CO2 emissions, investment & operating costs – to assess different technological setups for real-world central offices, taking into account the different scales and the existing infrastructure of the network nodes. Additionally, the designed optimization model should be critically analyzed regarding different scenarios of relevant future developments, e.g., w.r.t. renewable energy availability or energy price fluctuations. Finally, a scalable development strategy that can cope with the arising challenges for the central offices should be concluded, and the impact of different technological modifications on the central offices be quantified.
The use case is based on real data from the central offices of a large German telco company.
Student: Jan Hochgesand
Supervisor: Kirill Kuroptev
Time period: 11/21/2022 - 04/21/2023
Type: Pro-Seminars
This proseminar consists of literature/event research over power grid outages, explicitly focusing on cyber-attack-induced ones.The events should be clustered on their technical background and, in the literature, defined parameters like, e.g., loads not served, duration, and costs. Finally, a few relevant events, and their resulting possible countermeasures, should be studied in detail and comprehensively summarized.
Student: Hans Stenglein
Supervisor: Florian Steinke
Time period: 05/01/2023 - 10/31/2023
Type: Master Thesis
The flow of power in electricity networks depends on various stochastic factors, e.g. renewable energy feed-ins or time-varying loads. Their importance is recently growing with the introduction of more wind and sun to our energy systems. At the same time, however, the range of options to counteract the fluctuations is also growing, since more and more in-feeds become controllable, in addition to the traditional conventional generators, e.g., electric vehicle chargers of power-to-heat converters.
Grid operators have to ensure that the grid remains feasible under variations, i.e., that line flows, voltages, and the system frequency remain in an acceptable range. To this end, they can measure the grid’s state at different locations to influence some of the controllable in-feeds accordingly. We ask:
What is the minimal number of controllable in-feeds to influence and
the minimum number of measurement locations
such that the grid is guaranteed to remain in a feasible state with high probability?
To attack this control challenge, we first linearize the power flow equations. We also assume that the grid operator uses a linear feedback controller for setting the values of the controlled in-feeds given the measurements. The resulting linear constrained system [1] with stochastic inputs can be analyzed and optimized based on the so-called polynomial chaos expansion (PCE) [2]. The key idea of PCE is to interpret the involved random variables as functions and to decompose them along different functional components.
The proposed thesis shall formulate these points formally and numerically compute controllers for different example settings. A code toolbox for various steps will be provided. The work will require the knowledge and understanding of some probability/measure theory as well as numerical convex optimization techniques.
The thesis is to be jointly supervised by Prof. Dr. Timm Faulwasser (TU Dortmund) and Prof. Dr. Florian Steinke (TU Darmstadt).
[1] Edwin Mora: Minimal Admissible Control of Constrained Static Linear Systems with Applications to Power Systems, PhD Thesis, 2022, https://doi.org/https://doi.org/10.26083/tuprints-00020805
[2] Mühlpfordt, Tillmann, Timm Faulwasser, and Veit Hagenmeyer. "A generalized framework for chance-constrained optimal power flow." Sustainable Energy, Grids and Networks 16 (2018): 231-242.
Student: Isabella Nunes Grieser
Supervisor: Tobias Gebhard
Time period: 05/02/2023 - 10/31/2023
Type: Master Thesis
Human behavior can have significant impacts on energy systems, especially during crises. While there exist many models to describe energy demand, most of them do not focus on crisis events and neglect dependencies of human behavior. Therefore, changes in demand patterns can not be well predicted, which may lead to fatal consequences in crises. In this thesis, the modeling of these interconnections between humans and the connection of energy demand with other multimodal data shall be examined. Within a simulation model, dependencies between energy and other domains and the occurrence of critical effects are to be investigated. Further, the monitoring of system parameters and prevention of critical situations from a system perspective shall be tested.
Student: Konstantin Preusser
Supervisor: Allan Santos
Time period: 11/01/2022 - 01/24/2023
Type: Bachelor Thesis
This bachelor thesis aims to create an algorithm for the placement and control of phase-shifting transformers (PST) considering N-1 contingencies. The algorithm must guarantee grid feasibility for all realizations of the uncertain variables, such as renewable generation and variable loads, and be tractable for large use cases. The latter task is particularly challenging because (i) placement problems are usually solved using integer programming, which is NP-complete, and (ii) N-1 problems are combinatorial in nature. Grid simplification and scenario reduction approaches must be considered in the design of the algorithm, and a comparison with state-of-the-art placement algorithms must be carried out.
Student: Larissa Kunde
Supervisor: Tobias Gebhard
Time period: 05/17/2023 - 12/08/2023
Type:
Mini Forschung Der zeitliche Energieverbrauch von Haushalten wird von wesentlichen externen Faktoren beeinflusst, insbesondere der Jahres- und Tageszeit, sowie dem Wetter. Wenn diese Faktoren bekannt sind und herausgerechnet werden können, lassen sich darunterliegende Muster im Energieverbrauch, welche von menschlichem Verhalten geprägt sind, leichter analysieren. Unter anderem kann die Synchronisierung des Stromverbrauchs von Haushalten so genauer untersucht werden.
Aufgabenstellung
Im Rahmen dieses Miniprojekts soll ein umfassender Datensatz analysiert werden, welcher den Stromverbrauch von 38 Haushalten in hoher zeitlicher Auflösung über mehrere Jahre enthält. Zusätzlich sind Wetterdaten vorhanden, welche Informationen u.a. zu Temperatur und Sonneneinstrahlung enthalten.
Das Projekt umfasst die folgenden Arbeitspakte:
-Plausibilitätsanalyse der Zeitreihendaten der individuellen Haushaltslasten auf empirische Verteilungen, Strukturmuster und Anomalien
-Recherche von Methoden, den Einfluss externer Faktoren (z.B. Wetter) auf Energieverbrauch zu beschreiben
-Auswahl und Anwendung geeigneter Methoden, eventuelle Zusammenhänge externer Faktoren auf Energie- bzw. Stromverbrauch in dem genannten Datensatz zu untersuchen
-Untersuchung, ob und wie externe Faktoren mit der Synchronisierung der Haushalte korrelieren; Entwicklung eines Vorhersagemodells
Als Abgabe wird ein lauffähiger und verständlicher Programmcode, inklusive der relevanten Datengrundlage erwartet. Die Prüfungsleistung wird durch eine Abschlusspräsentation erbracht.
Student: Joao Kroeger
Supervisor: Allan Santos
Time period: 03/01/2023 - 08/31/2023
Type: Master Thesis
Smart grids are key components for enabling more flexible, cleaner and more robust energy systems. However, the real-time control of thousands of active elements such as distributed energy resources, batteries, and charging stations for electric vehicles is challenging. Grid operation relies on solving several optimization problems. One of them is the optimal power flow (OPF), whose goal is to find the operating point of all elements of the grid such that the power production cost is minimized while operation constraints are met. In legacy, static grids, OPF has to be solved every couple of minutes. On the other hand, the control of smart grids requires solving OPF problems with thousandfold more variables and on the order of seconds.
In this thesis, new methods for the speed-up of the OPF calculation are going to be investigated. Special focus will be given on using graph neural networks (GNN) for the development of surrogates. GNN is a special neural network architecture that is suited for learning on graph-like structures by leveraging non-Euclidian properties. This thesis also aims at developing zero-shot learning algorithms, i.e., methods that are able to solve the OPF with high accuracy not only for the grid from which training data was generated, but also on modified versions of it, e.g. the addition of new photovoltaic panels.